Process monitoring requires an efficient and accurate alarm when the process is in an abnormal status. The Bayesian analysis facilitates the real-time update of the process status in a probabilistic view, which provides rich information/evidence for decision-making.
Example: Contamination source identification in a water distribution network
Efficient identification of the source of contamination in a water distribution network is crucial to the safe operation of the system. However, the water demands at different locations in the water distribution network are stochastic, which makes the flow rates also stochastic. This makes the tracking and identification of the contamination source a challenging task.
A real water distribution network is shown in Figure 4, where the contamination can happen at any of the 126 nodes (locations). Only five sensors are available in the network to periodically report the binary contamination information (contamination happens at the sensor location or not). The contamination monitoring and source identification must be processed online.
The Bayesian analysis can help monitor the contamination situations in each location and identify where is the source of the contamination, using the sequentially reported data from the five sensors. The larger the points in Figure 4, the higher possibility the contamination can be successfully identified.
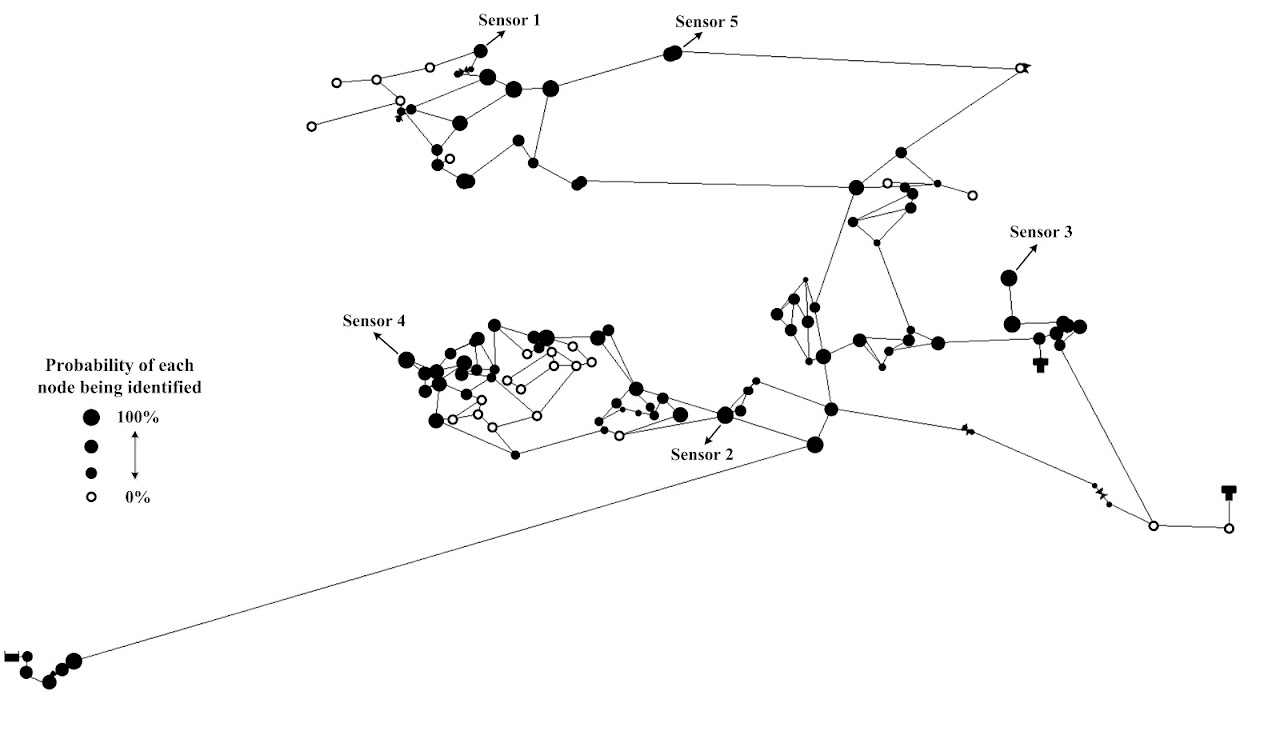
Figure 4. Contamination source identification in a water distribution network.